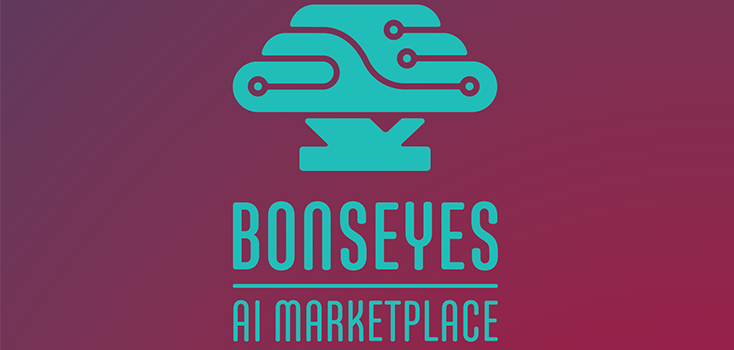
Use case: Evidence Based Medicine Demonstrator
Today ordinary medical treatment is mostly based on personal expertise and experience of physicians, little to no automated technical support is given to neither physician nor patient. No autonomous actions are carried out to support the medical routine or reduce the workload and no automatic hints are given to improve the treatment by employing knowledge obtained from prior cases with similar indications. However, such tasks could be facilitated by Artificial Intelligence (AI)-Systems and would allow the medical staff to spend more time on actual patient care.
In general, a lot of data is collected and saved during the stay and the treatment of a patient. It ranges from the basic patient’s information (name, gender, age etc.) to detected health conditions and ongoing treatments – all collected via their medical history to specific diagnostic findings of different departments. Medical staff therefore spends a huge part of their time on non-care related tasks such as documenting and registering the acquired information in the IT-systems, which can also exist on many different systems or departments.
However, a centralized hub and connection is not given in most instances, therefore inefficient comparisons or double record keeping is often standard and hinders a helpful supporting data framework for the staff. In that context, data can get lost, is overseen or saved multiple times in different versions, it might also not be accessible when necessary. In consequence treatments may are prolonged, workflows are disturbed and the patients safety might be affected as well.
Problems in data sharing and possible solutions
Because of inefficient data management multiple problems that have been detected:
- Inefficient workflows that result of fragmented knowledge sharing
- Less time spent on treatment, because of non-care related tasks
- Imprecise documentation
- Providing unnecessary treatment
- Causing avoidable harm to patient
Therefore, streamlining clinical processes would both reduce workload and safe time and costs at once. In this regard, it is advisable, to develop a system, that is able to assist caregivers during their clinical work routine in order to reduce workload, increase patient safety and thereby reduce healthcare costs.
A couple of goals have been defined that for a successful solution including:
- Improving the Quality of documentation and effort reduction
- Streamlining workflows, reducing workload for providers
- Simplifying the AI systems development for hospitals
To reach these goals, “Bonseyes” aims to provide technical infrastructure and resources, while accelerating the design and programming of artificial intelligence systems by 50%. Another part is to reduce the complexity in training models for distributed embedded systems. By the adoption of Bonseyes on a wide scale, external developers can be invited to contribute based on the provided data and improve existing models or even generate new solutions. Therefore the intermediate goal for the near future is to support health care providers at least during treatment with automation of uncritical or non-care related tasks.
Evidence-Based-Medicine and the challenges within the use case:
Evidence-based-medicine (EBM) is the vision called, that plans to adopt IT and further digitalisation in healthcare. Since Electronic health records (EHRs) and any other form of digital health information constitute the basis of automatic support systems the intermediate goal for the near future is to support health care providers at least during treatment with automation of uncritical or non-care related tasks. The secure collection, processing and labelling of sensitive data as well as the consecutive generation of models will be facilitated by the Bonseyes platform. Therefore, very large dataset will be needed to account for the diverse physiology of patients.
Two challenges present themselves, when addressing the issue and the envisioned support systems will be described in detail in the following section.
Challenge 1: Automated Transcription of Patient Interviews based on Voice Recording for Safety Checks
The long-term goal is the creation of the Evidence Based Medicine System by the utilization of AI. Hereby smart services will assist healthcare providers during their clinical work in order to increase patient safety, reduce workload and improve documentation and data quality within EHRs.
In this regard, the Diagnosis Speech Recognition System (DSR-System) will function as a tool for data collection and corresponding safety checks during the outpatient diagnosis and treatment. When arriving in the outpatient department the patient is registered equipped with an embedded device for data collection during their hospitalization. The device capable of recording audio and either perform edge-computation or transferring it to a server via FIWARE for further computation, the information extracted from the voice recognition will be available in real-time and to every staff member involved in the treatment process.
Challenge 2: Surgical Workflow Recognition for collaborative Operation Theatre
The Surgical Workflow Recognition System gathers available data sources in the OR, interprets them and derives certain actions and therefore is enhanced by artificial intelligence to evolve towards a collaborative working environment. Based on image recognition on the laparoscopic video data, the system will classify the different phases of a predefined surgery. An underlying process model of the surgery will help to evaluate the current surgical step in perspective of the overall course of surgery. Based on a reliable detection of surgical phases, assistive functions for the surgical team can be anticipated and adequate actions may be performed. The actions could also be structured in different “levels of criticality” which are supposed to account for the possible effects of the actions on the overall outcome of the procedure.